|
 |
Publications
|
2025
- H. Espino and J. L. Krichmar, "Vector-Based Navigation Inspired by Directional Place Cells," in From Animals to Animats 17, O. Brock and J. Krichmar, Eds., 20252025, vol. 14993: Lecture Notes in Artificial Intelligence, Springer Nature, pp. 27-38.[pdf]
- S. A. Mohaddesi, M. Hegarty, E. R. Chrastil, and J. L. Krichmar, "Benefit of Varying Navigation Strategies in Robot Teams," in From Animals to Animats 17, O. Brock and J. Krichmar, Eds., 2025, vol. 14993: Lecture Notes in Artificial Intelligence, Springer Nature, pp. 63-77.[pdf]
- H. Pae and J. L. Krichmar, "Integrated Information in Genetically Evolved Braitenberg Vehicles," in From Animals to Animats 17, O. Brock and J. Krichmar, Eds., 2025, vol. 14993: Lecture Notes in Artificial Intelligence, Springer Nature, pp. 236-247.[pdf]
- M. A. Pfeiffer et al., "CuttleBot: Emulating Cuttlefish Behavior and Intelligence in a Novel Robot Design," in From Animals to Animats 17, Irvine, O. Brock and J. Krichmar, Eds., 2025, vol. 14993: Lecture Notes in Artificial Intelligence, Springer Nature, pp. 93-105.[pdf]
2024
- Alonso, N., & Krichmar, J. L. (2024). A sparse quantized hopfield network for online- continual memory. Nature Communications, 15(1), 3722. https://doi.org/10.1038/s41467- 024-46976-4.[pdf]
- Alonso, N., Krichmar, J., & Neftci, E. (2024). Understanding and Improving Optimization in Predictive Coding Networks. Proceedings of the AAAI Conference on Artificial Intelligence, 38(10), 10812-10820. https://doi.org/10.1609/aaai.v38i10.28954.[pdf]
- H. Espino, R. Bain, and J. L. Krichmar, "A Rapid Adapting and Continual Learning Spiking Neural Network Path Planning Algorithm for Mobile Robots," IEEE Robotics and Automation Letters, vol. 9, no. 11, pp. 9542-9549, 2024, doi: 10.1109/LRA.2024.3457371.[pdf][website]
- H.G. Pan, T. Weiss, S. A. Mohaddesi, J. W. Szura, and J. L. Krichmar, "Navigation and Cognitive Load in Telepresence Robots," in 2024 IEEE International Conference on Development and Learning (ICDL), 20-23 May 2024 2024, pp. 1-6, doi: 10.1109/ICDL61372.2024.10644592.[pdf]
2023
- Ahumada-Newhart, V., Kashyap, H. J., Hwu, T., Tian, Y., Mirzakhanian, L., Minton, M., Seader, S., Hedden, S., Moore, D., Krichmar, J., & Eccles, J. S. (2023). Evaluation of the Toyota Human Support Robot (HSR) for Social Interaction and Learning. The International Journal of Technology, Knowledge, and Society, 19(1), 21-52. https://doi.org/doi:10.18848/1832-3669/CGP/v19i01/21-52 [pdf]
- Balaji, A., Huynh, P. K., Catthoor, F., Dutt, N. D., Krichmar, J. L., & Das, A. (2023). NeuSB: A Scalable Interconnect Architecture for Spiking Neuromorphic Hardware. IEEE Transactions on Emerging Topics in Computing, 11(2), 373-387. https://doi.org/10.1109/Tetc.2023.3238708 [pdf]
- Chen, K. X., Kashyap, H. J., Krichmar, J. L., & Li, X. M. (2023). What can computer vision learn from visual neuroscience? Introduction to the special issue. Biological Cybernetics, 117(4-5), 297-298. https://doi.org/10.1007/s00422-023-00977-6 [pdf]
- Espino, H., Bain, R., & Krichmar, J. L. (2023). Selective Memory Replay Improves Exploration in a Spiking Wavefront Planner. 2023 International Joint Conference on Neural Networks (IJCNN), DOI: 10.1109/IJCNN54540.2023.10191940 [pdf]
- Kopsick, J. D., Tecuatl, C., Moradi, K., Attili, S. M., Kashyap, H. J., Xing, J., Chen, K., Krichmar, J. L., & Ascoli, G. A. (2023). Robust Resting-State Dynamics in a Large-Scale Spiking Neural Network Model of Area CA3 in the Mouse Hippocampus. Cognit Comput, 15(4), 1190-1210. https://doi.org/10.1007/s12559-021-09954-2 [pdf]
- Krichmar, J. L., & He, C. (2023). Importance of Path Planning Variability: A Simulation Study [https://doi.org/10.1111/tops.12568]. Topics in Cognitive Science, 15(1), 139-162. https://doi.org/https://doi.org/10.1111/tops.12568 [pdf]
- Niedermeier, L., & Krichmar, J. L. (2023). Experience-Dependent Axonal Plasticity in Large-Scale Spiking Neural Network Simulations. 2023 International Joint Conference on Neural Networks (IJCNN), DOI: 10.1109/IJCNN54540.2023.10191241 [pdf]
- Xing, J., Nagata, T., Zou, X., Neftci, E., & Krichmar, J. L. (2023). Achieving efficient interpretability of reinforcement learning via policy distillation and selective input gradient regularization. Neural Networks, 161, 228-241. https://doi.org/https://doi.org/10.1016/j.neunet.2023.01.025 [pdf]
2022
- Xing, J., Chrastil, E.R., Nitz, D.A., and Krichmar, J.L. (2022). Linking global top-down views to first-person views in the brain. Proceedings of the National Academy of Sciences 119, e2202024119. 10.1073/pnas.2202024119.[pdf]
- Alonso, N., Millidge, B., Krichmar, J., & Neftci, E. (2022). A Theoretical Framework for Inference Learning. Advances in Neural Information Processing Systems, Eds. Alice H. Oh, Alekh Agarwal, Danielle Belgrave, and Kyunghyun Cho. https://openreview.net/forum?id=7yJMZwhIC2k." href="alonso-inference-learning-neurips2022.pdf">[pdf]
- Krichmar, J.L., Ketz, N.A., Pilly, P.K., and Soltoggio, A. (2022). Flexible Path Planning in a Spiking Model of Replay and Vicarious Trial and Error. In L. Cañamero, P. Gaussier, M. Wilson, S. Boucenna, and N. Cuperlier, eds. From Animals to Animats 16 - Simulation of Adaptive Behavior. Springer International Publishing. pp. 177-189.[pdf]
- Niedermeier, L., Chen, K., Xing, J., Das, A., Kopsick, J., Scott, E., Sutton, N., Weber, K., Dutt, N., and Krichmar, J.L. (2022). CARLsim 6: An Open Source Library for Large-Scale, Biologically Detailed Spiking Neural Network Simulation. 2022 International Joint Conference on Neural Networks (IJCNN).[pdf]
- Burachas, G., Grigsby, S., Ferguson, B., Krichmar, J., and Rao, R. (2022). Metacognitive Mechanisms for Novelty Processing: Lessons for AI. Paper presented at: AAAI Spring Symposium.[pdf]
- Chen, K., Beyeler, M., and Krichmar, J.L. (2022). Cortical Motion Perception Emerges from Dimensionality Reduction with Evolved Spike-Timing-Dependent Plasticity Rules. The Journal of Neuroscience 42, 5882.[pdf]
- Hwu, T.J., and Krichmar, J.L. (2022). Neurorobotics: Neuroscience and Robots. In Cognitive Robotics, A. Cangelosi, and M. Asada, eds. (The MIT Press), pp. 19-40.[pdf]
- Krichmar, J.L., and Hwu, T.J. (2022). Design Principles for Neurorobotics. Front Neurorobotics 16.[pdf]
- Kudithipudi, D., Aguilar-Simon, M., Babb, J., Bazhenov, M., Blackiston, D., Bongard, J., Brna, A.P., Chakravarthi Raja, S., Cheney, N., Clune, J., et al. (2022). Biological underpinnings for lifelong learning machines. Nature Machine Intelligence 4, 196-210.[pdf]
- Titirsha, T., Song, S., Das, A., Krichmar, J., Dutt, N., Kandasamy, N., and Catthoor, F. (2022). Endurance-Aware Mapping of Spiking Neural Networks to Neuromorphic Hardware. IEEE Transactions on Parallel and Distributed Systems 33, 288-301.[pdf]
2021
- Krichmar, J.L., and He, C. (2021). Importance of Path Planning Variability: A Simulation Study. Topics in Cognitive Science, DOI: https://doi.org/10.1111/tops.12568.[pdf]
- Krichmar, J.L. (2021). Gerald Edelman’s Steps Toward a Conscious Artifact. Journal of Artificial Intelligence and Consciousness, 1-9. DOI: https://doi.org/10.1142/S2705078521500144[pdf]
- Krichmar, J.L., Olds, J.L., Sanchez-Andres, J.V., and Tang, H. (2021). Editorial: Explainable Artificial Intelligence and Neuroscience: Cross-Disciplinary Perspectives. Front Neurorobotics 15.[pdf]
- Kashyap, H.J., Fowlkes, C.C., and Krichmar, J.L. (2021). Sparse Representations for Object- and Ego-Motion Estimations in Dynamic Scenes. IEEE Transactions on Neural Networks and Learning Systems 32, 2521-2534.[pdf]
- Chen, K., Johnson, A., O., S.E., Xinyun, Z., D., D.J.K., A., N.D., and L., K.J. (2021). Differential Spatial Representations in Hippocampal CA1 and Subiculum Emerge in Evolved Spiking Neural Networks. Paper presented at: International Joint Conference on Neural Networks (IJCNN).[pdf]
- Xing, J., Nagata, T., Zou, X., Neftci, E., and Krichmar, J.L. (2021). Domain Adaptation In Reinforcement Learning Via Latent Unified State Representation. In Proceedings of the AAAI Conference on Artificial Intelligence, pp. 10452-10459.[pdf]
- Zou, X., Scott, E.O., Johnson, A.B., Chen, K., Nitz, D.A., Jong, K.A.D., and Krichmar, J.L. (2021). Neuroevolution of a Recurrent Neural Network for Spatial and Working Memory in a Simulated Robotic Environment. Paper presented at: Genetic and Evolutionary Computation Conference (GECCO) (Lille, France: ACM).[pdf][arXiv version]
2020
- Chen, K., Hwu, T., Kashyap, H.J., Krichmar, J.L., Stewart, K., Xing, J., and Zou, X. (2020). Neurorobots as a Means Toward Neuroethology and Explainable AI. Front Neurorobotics, 14. doi: 10.3389/fnbot.2020.570308.[pdf]
- Chiba, A. A. and J. L. Krichmar (2020). "Neurobiologically Inspired Self-Monitoring Systems." Proceedings of the IEEE 108(7): 976-986.[pdf]
- Hwu, T., and Krichmar, J.L. (2020). A neural model of schemas and memory encoding. Biological Cybernetics, 114, 169-186.10.1007/s00422-019-00808-7.[pdf]
- Zou, X., Hwu, T., Krichmar, J., and Neftci, E. (2020). Terrain Classification with a Reservoir-Based Network of Spiking Neurons. Paper presented at: 2020 IEEE International Symposium on Circuits and Systems (ISCAS). pp. 1-5. doi: 10.1109/ISCAS45731.2020.9180740.[pdf]
- Kashyap, H.J., Fowlkes, C.C., and Krichmar, J.L. (2020). Sparse Representations for Object- and Ego-Motion Estimations in Dynamic Scenes. IEEE Transactions on Neural Networks and Learning Systems, 1-14.[pdf]
- Hwu, T., Kashyap, H.J., and Krichmar, J.L. (2020). A Neurobiological Schema Model for Contextual Awareness in Robotics. Paper presented at: IEEE International Joint Conference on Neural Networks (IJCNN) (Glasgow). Winner! Best paper award. [pdf][video presentation]
- Xing, J., Zou, X., and Krichmar, J.L. (2020). Neuromodulated Patience for Robot and Self-Driving Vehicle Navigation. Paper presented at: IEEE International Joint Conference on Neural Networks (IJCNN) (Glasgow).[pdf][video presentation]
- Balaji, A., Adiraju, P., Kashyap, H.J., Das, A., Krichmar, J.L., Dutt, N.D., and Catthoor, F. (2020). PyCARL: A PyNN Interface for Hardware-Software Co-Simulation of Spiking Neural Network. Paper presented at: IEEE International Joint Conference on Neural Networks (IJCNN) (Glasgow).[pdf]
- Balaji, A., Das, A., Wu, Y., Huynh, K., Dell'Anna, F.G., Indiveri, G., Krichmar, J.L., Dutt, N.D., Schaafsma, S., and Catthoor, F. (2020). "Mapping Spiking Neural Networks to Neuromorphic Hardware." IEEE Transactions on Very Large Scale Integration (Vlsi) Systems 28(1): 76-86.[pdf]
- Belkaid, M. and J. L. Krichmar (2020). "Modeling uncertainty-seeking behavior mediated by cholinergic influence on dopamine." Neural Networks, 125: 10-18.[pdf]
- Zou, X., Kolouri, S., Pilly, P.K., and Krichmar, J.L. (2020). Neuromodulated attention and goal-driven perception in uncertain domains. Neural Networks 125, 56-69.[pdf]
2019
- Xing, J., Zou, X., and Krichmar, J.L. (2019). Neuromodulated Patience for Robot and Self-Driving Vehicle Navigation. arXiv:190906533 [cs.RO][pdf]
- Zou, X., Pilly, P.K., Kolouri, S., and Krichmar, J.L. (2019). Neuromodulated Goal-Driven Perception in Uncertain Domains. arXiv:190300068 [cs.NE].[pdf]
- Beyeler, M., Rounds, E.L., Carlson, K.D., Dutt, N., and Krichmar, J.L. (2019). Neural correlates of sparse coding and dimensionality reduction. PLoS Comput Biol 15, e1006908.[pdf]
- Krichmar, J.L., Severa, W., Khan, M.S., and Olds, J.L. (2019). Making BREAD: Biomimetic Strategies for Artificial Intelligence Now and in the Future. Frontiers in Neuroscience 13.[pdf]
- Krichmar, J.L., Hwu, T., Zou, X., Hylton, T. (In Press). Advantage of prediction and mental imagery for goal-directed behaviour in agents and robots. Cognitive Computation and Systems.[pdf]
- Gaussier, P., Banquet, J.P., Cuperlier, N., Quoy, M., Aubin, L., Jacob, P.-Y., Sargolini, F., Save, E., Krichmar, J.L., and Poucet, B. (2019). Merging information in the entorhinal cortex: what can we learn from robotics experiments and modeling? The Journal of Experimental Biology, 222:1-13.[pdf]
2018
- Chou*, T.-S., Kashyap*, H.J., Xing, J., Listopad, S., Rounds, E.L., Beyeler, M., Dutt, N., and Krichmar, J.L. (2018). CARLsim 4: An Open Source Library for Large Scale, Biologically Detailed Spiking Neural Network Simulation using Heterogeneous Clusters. Paper presented at: International Joint Conference on Neural Networks (IJCNN) (Rio De Janeiro: IEEE). (*co-first authors) (Finalist for the best student paper award.) [pdf]
- Hwu, T., Wang, A.Y., Oros, N., and Krichmar, J.L. (2018). Adaptive Robot Path Planning Using a Spiking Neuron Algorithm With Axonal Delays. IEEE Transactions on Cognitive and Developmental Systems 10, 126-137. (Winner! Best paper award.) [pdf]
- Kashyap, H.J., Detorakis, G., Dutt, N., Krichmar, J.L., and Neftci, E. (2018). A Recurrent Neural Network Based Model of Predictive Smooth Pursuit Eye Movement in Primates. Paper presented at: International Joint Conference on Neural Networks (IJCNN) (Rio De Janeiro: IEEE).[pdf]
- Krichmar, J.L. (2018). Neurorobotics—A Thriving Community and a Promising Pathway Toward Intelligent Cognitive Robots. Frontiers in neurorobotics 12.[weblink]
- Rounds, E.L., Alexander, A.S., Nitz, D.A., and Krichmar, J.L. (2018). Conjunctive coding in an evolved spiking model of retrosplenial cortex. Behav Neurosci. 1. DOI: 10.1037/bne0000236.[pdf]
- Tang, H., Huang, T., Krichmar, J.L., Orchard, G., and Basu, A. (2018). Guest Editorial Special Issue on Neuromorphic Computing and Cognitive Systems. IEEE Transactions on Cognitive and Developmental Systems 10, 122-125.[pdf]
- Krichmar, J.L., and Chou, T.-S. (2018). A Tactile Robot for Developmental Disorder Therapy. Paper presented at: Technology, Mind, and Society (Washington, DC: ACM).[pdf]
- Venkadesh, S., Komendantov, A.O., Listopad, S., Scott, E.O., De Jong, K., Krichmar, J.L., and Ascoli, G.A. (2018). Evolving Simple Models of Diverse Intrinsic Dynamics in Hippocampal Neuron Types. Frontiers in Neuroinformatics 12.[pdf]
- Das, A., Pradhapan, P., Groenendaal, W., Adiraju, P., Rajan, R.T., Catthoor, F., Schaafsma, S., Krichmar, J.L., Dutt, N., and Van Hoof, C. (2018). Unsupervised heart-rate estimation in wearables with Liquid states and a probabilistic readout. Neural Netw 99, 134-147.[weblink]
2017
- Avery, M.C., and Krichmar, J.L. (2017). Neuromodulatory Systems and Their Interactions: A Review of Models, Theories, and Experiments.[pdf]
- Craig, A.B., Grossman, E., and Krichmar, J.L. (2017). Investigation of autistic traits through strategic decision-making in games with adaptive agents. Scientific Reports 7, 5533, DOI:10.1038/s41598-017-05933-6.[pdf][web link]
- Hwu, T., Isbell, J., Oros, N., and Krichmar, J. (2017a). A self-driving robot using deep convolutional neural networks on neuromorphic hardware. Paper presented at: 2017 International Joint Conference on Neural Networks (IJCNN), pp. 635-641.[pdf]
- Hwu, T., Krichmar, J.L., and Zou, X. (2017b). A Complete Neuromorphic Solution to Outdoor Navigation and Path Planning. Paper presented at: IEEE International Symposium on Circuits & Systems (ISCAS) (Baltimore, MD), pp. 2707-2710.[pdf]
- Oess, T., Krichmar, J.L., and Röhrbein, F. (2017). A Computational Model for Spatial Navigation Based on Reference Frames in the Hippocampus, Retrosplenial Cortex, and Posterior Parietal Cortex. Frontiers in Neurorobotics 11.[pdf]
2016
- Hwu, T., Isbell, J., Oros, N., and Krichmar, J. (2016). A Self-Driving Robot Using Deep Convolutional Neural Networks on Neuromorphic Hardware. arXiv arXiv:1611.01235 [cs.NE].[pdf]
- Beyeler, M., Dutt, N., and Krichmar, J.L. (2016). 3D Visual Response Properties of MSTd Emerge from an Efficient, Sparse Population Code. The Journal of Neuroscience 36, 8399-8415.[pdf]
- Rounds, E.L., Scott, E.O., Alexander, A.S., De Jong, K.A., Nitz, D.A., and Krichmar, J.L. (2016). An Evolutionary Framework for Replicating Neurophysiological Data with Spiking Neural Networks. Paper to be presented at the 14th International Conference of Parallel Problem Solving from Nature (PPSN). Edinburgh, Scotland.[pdf]
- Krichmar, J.L. (2016). Path Planning using a Spiking Neuron Algorithm with Axonal Delays. Paper Presented at the 2016 IEEE Congress on Evolutionary Computation. Vancouver. pp. 1219-1226[pdf][spikeWaveSourceCode][mapGeneratorCode]
- Craig, A.B., Phillips, M.E., Zaldivar, A., Bhattacharyya, R., and Krichmar, J.L. (2016). Investigation of biases and compensatory strategies using a probabilistic variant of the Wisconsin Card Sorting Test. Frontiers in Psychology 7:17.[pdf]
2015
- Beyeler, M., Oros, N., Dutt, N., and Krichmar, J.L. (2015). A GPU-accelerated cortical neural network model for visually guided robot navigation. Neural Networks, 72, 75-87.[pdf]
- Krichmar, J.L., Conradt, J., and Asada, M. (2015). Neurobiologically Inspired Robotics: Enhanced Autonomy through Neuromorphic Cognition. Neural Networks, 72, 1-2.[pdf]
- Asher, D.E., Oros, N., and Krichmar, J.L. (2015). The Importance of Lateral Connections in the Parietal Cortex for Generating Motor Plans. PLoS ONE 10, e0134669.[pdf]
- Beyeler, M.*, Carlson, K.D.*, Chou, T.-S.*, Dutt, N., and Krichmar, J.L. (2015). CARLsim 3: A User-Friendly and Highly Optimized Library for the Creation of Neurobiologically Detailed Spiking Neural Networks. Paper presented at: International Joint Conference on Neural Networks (Killarney, Ireland: IEEE Explore). (*co-first authors)[pdf]
- Chou, T.-S., Bucci, L.D., and Krichmar, J.L. (2015). Learning Touch Preferences with a Tactile Robot Using Dopamine Modulated STDP in a Model of Insular Cortex. Frontiers in Neurorobotics 9.[pdf]
- Avery, M., and Krichmar, J.L. (2015). Improper activation of D1 and D2 receptors leads to excess noise in prefrontal cortex. Frontiers in Computational Neuroscience Vol. 9, Article 31, 1-15.[pdf]
- Krichmar, J.L., Coussy, P., and Dutt, N. (2015). Large-Scale Spiking Neural Networks using Neuromorphic Hardware Compatible Models. ACM Journal on Emerging Technologies in Computing Systems, Vol. 11, No. 4, Article 36 , 1-18.[pdf]
2014
- Zaldivar, A., and Krichmar, J.L. (2014). Allen Brain Atlas-Driven Visualizations: A Web-Based Gene Expression Energy Visualization Tool. Frontiers in Neuroinformatics 8.[pdf]
- Bucci, L.D., Chou, T.-s., and Krichmar, J.L. (2014). Tactile Sensory Decoding in a Neuromorphic Interactive Robot. Paper presented at: 2014 IEEE Conference on Robotics & Automation (Hong Kong).[pdf]
- Asher, D.E., Krichmar, J.L., and Oros, N. (2014). Evolution of Biological Plausible Neural Networks Performing a Visually Guided Reaching Task. Paper presented at: Genetic and Evolutionary Computation Conference (GECCO) (Vancouver: ACM).[pdf]
- Carlson, K.D., Nageswaran, J.M., Dutt, N., and Krichmar, J.L. (2014). An efficient automated parameter tuning framework for spiking neural networks. Frontiers in Neuroscience 8(10). [pdf]
- Beyeler, M., Richert, M., Dutt, N.D., and Krichmar, J.L. (2014). Efficient spiking neural network model of pattern motion selectivity in visual cortex. Neuroinformatics. [pdf]
- Avery, M.C., Dutt, N., and Krichmar, J.L. (2014). Mechanisms underlying the basal forebrain enhancement of top-down and bottom-up attention. The European journal of neuroscience 39, 852-865.[pdf]
- Oros, N., Chiba, A.A., Nitz, D.A., Krichmar, J.L. (2014). Learning to ignore - A modeling study of the decremental cholinergic pathway and its influence on attention and learning. Learning and Memory, 21: 105-118 [pdf]
- Carlson, K.D., Beyeler, M., Dutt, N., and Krichmar, J.L. (2014). GPGPU Accelerated Simulation and Parameter Tuning for Neuromorphic Applications. Proceedings of the 19th Asia and South Pacific Design Automation Conference (ASP-DAC'14). [pdf]
2013
- Asher*, D.A., Craig*, A.B., Zaldivar*, A., Brewer, A.A., and Krichmar, J.L. (2013). A dynamic, embodied paradigm to investigate the role of serotonin in cost and decision-making. Frontiers in Integrative Neuroscience 7(78). (*co-first authors) [pdf]
- Zaldivar, A., and Krichmar, J. (2013). Interactions between the neuromodulatory systems and the amygdala: exploratory survey using the Allen Mouse Brain Atlas. Brain Structure and Function, 218, 1513-1530.[pdf]
- Carlson, K.D., Richert, M., Dutt, N., and Krichmar, J.L. (2013). Biologically Plausible Models of Homeostasis and STDP: Stability and Learning in Spiking Neural Networks. Paper presented at: International Joint Conference on Neural Networks (Dallas, TX: IEEE Explore).[pdf]
- Krichmar, J.L., and Rohrbein, F. (2013). Value and Reward Based Learning in Neurorobots. Frontiers in neurorobotics 7.[pdf]
- Beyeler, M., Dutt, N.D., and Krichmar, J.L. (2013). Categorization and decision-making in a neurobiologically plausible spiking network using a STDP-like learning rule. Neural Networks 48, 109-124.[pdf]
- Craig, A.B., Asher, D.E., Oros, N., Brewer, A.A., and Krichmar, J.L. (2013). Social contracts and human-computer interaction with simulated adapting agents. Adaptive Behavior 21, 371-387.[pdf]
- Krichmar, J.L. (2013). A neurorobotic platform to test the influence of neuromodulatory signaling on anxious and curious behavior. Frontiers in neurorobotics 7, 1-17.[pdf]
2012
- Oros, N., and Krichmar, J.L. (2012). Neuromodulation, Attention and Localization Using a Novel Android™ Robotic Platform. In IEEE International Conference on Development and Learning and Epigenetic Robotics (IEEE ICDL-EpiRob 2012) (San Diego, CA).[pdf]
- Chelian, S.E., Oros, N., Zaldivar, A., Krichmar, J., and Bhattacharyya, R. (2012). Model of the interactions between neuromodulators and prefrontal cortex during a resource allocation task. In IEEE International Conference on Development and Learning and Epigenetic Robotics (IEEE ICDL-EpiRob 2012) (San Diego, CA).[pdf]
- Asher, D.E., Zaldivar, A., Barton, B., Brewer, A.A., and Krichmar, J.L. (2012). Reciprocity and Retaliation in Social Games With Adaptive Agents. IEEE Transactions on Autonomous Mental Development 4, 226-238.[pdf]
- Krichmar, J.L. (2012).Design principles for biologically inspired cognitive robotics. Biologically Inspired Cognitive Architectures 1, 73-81.[pdf]
- Asher, D.E., Zhang, S., Zaldivar, A., Lee, M.D., and Krichmar, J.L. (2012). Modeling individual differences in socioeconomic game playing. Paper presented at: COGSCI 2012 - The Annual Meeting of the Cognitive Science Society (Sapporo, Japan).[pdf]
- Avery, M., Krichmar, J.L., and Dutt, N. (2012). Spiking Neuron Model of Basal Forebrain Enhancement of Visual Attention. Paper presented at: IEEE World Congress on Computational Intelligence (Brisbane, Australia).[pdf]
- Krichmar, J.L. (2012). A Biologically Inspired Action Selection Algorithm Based on Principles of Neuromodulation. Paper presented at: IEEE World Congress on Computational Intelligence (Brisbane, Australia).[pdf]
- Avery, M.C., Nitz, D.A., Chiba, A.A., and Krichmar, J.L. (2012). Simulation of Cholinergic and Noradrenergic Modulation of Behavior in Uncertain Environments. Frontiers in Computational Neuroscience 6, 1-16.[pdf]
2011
- Krichmar, J.L., and Wagatsuma, H. (2011). Neuromorphic and Brain-Based Robots. In Biologically Inspired Cognitive Architectures, A.V. Samsonovich, and K.R. Johannsdottir, eds. (IOS Press), pp. 209-214.[pdf]
- Krichmar, J.L., Dutt, N., Nageswaran, J.M., and Richert, M. (2011). Neuromorphic Modeling Abstractions and Simulation of Large-Scale Cortical Networks. Paper presented at: IEEE/ACM International Conference on Computer-Aided Design (ICCAD) (San Jose, CA).[pdf]
- Richert, M., Nageswaran, J.M., Dutt, N., and Krichmar, J.L. (2011). An efficient simulation environment for modeling large-scale cortical processing. Frontiers in Neuroinformatics 5, 1-15.[pdf][sourcecode]
2010
- Nageswaran, J.M., Richert, M., Dutt, N., and Krichmar, J.L. (2010). Towards reverse engineering the brain: Modeling abstractions and simulation frameworks. Paper presented at: VLSI System on Chip Conference (VLSI-SoC), 2010 18th IEEE/IFIP.[pdf]
- Zaldivar, A., Asher, D.E., and Krichmar, J.L. (2010). Simulation of How Neuromodulation Influences Cooperative Behavior. In Simulation of Adaptive Behavior: From Animals to Animats, S. Doncieux, J.-A. Meyer, A. Guillot, and J. Hallam, eds. (Berlin Heidelberg, Springer-Verlag Lecture Notes on Artificial Intelligence (LNAI 6226)), pp. 649-660.[pdf]
- Asher, D.E., Zaldivar, A., and Krichmar, J.L. (2010). Effect of Neuromodulation on Performance in Game Playing: A Modeling Study. Paper presented at: 2010 IEEE 9th International Conference on Development and Learning (Ann Arbor, Michigan, IEEE Xplore).[pdf]
2009
- Nageswaran, J.M., Dutt, N., Krichmar, J.L., Nicolau, A., and Veidenbaum, A.V. (2009). A configurable simulation environment for the efficient simulation of large-scale spiking neural networks on graphics processors. Neural Networks 22, 791-800.[ScienceDirect-pdf]
- Cox BR, Krichmar JL. (2009) Neuromodulation as a Robot Controller: A Brain Inspired Design Strategy for Controlling Autonomous Robots. IEEE Robotics & Automation Magazine September 2009. [pdf] [see CARL-1 videos]
- Moorkanikara Nageswaran, J., Dutt, N., Krichmar, J.L., Nicolau, A., and Veidenbaum, A.
(2009). Efficient Simulation of Large-Scale Spiking Neural Networks Using CUDA
Graphics Processors Paper presented at: IJCNN (Atlanta, GA)[pdf]
2008
- Krichmar, J.L. (2008). The Neuromodulatory System - A Framework for Survival and Adaptive Behavior in a Challenging World. Adaptive Behavior, 16, 385-399. [pdf]
- McKinstry, J.L., Seth, A.K., Edelman, G.M., and Krichmar, J.L. (2008). Embodied Models of Delayed Neural Responses: Spatiotemporal Categorization and Predictive Motor Control in Brain Based Devices. Neural Networks 21, 553-561. [pdf]
2007
- J.G. Fleischer and J.L. Krichmar (2007). Sensory integration and remapping in a medial temporal lobe model during maze navigation by a brain-based device. Journal of Integrative Neuroscience, 6(3):403-431. [pdf]
- J.G. Fleischer, J.A. Gally, G.M.
Edelman, and J.L. Krichmar (2007) Retrospective and prospective
responses arising in a modeled hippocampus during maze navigation
by a brain-based device. Proc Nat Acad Sci USA 104(9):3556-3561. [pdf]
2006
- J.L. Krichmar and G.M. Edelman (2006). Principles Underlying the Construction of Brain-Based Devices, In Adaptation in Artificial and Biological Systems, T. Kovacs, and J. A. R. Marshall, eds. (Bristol UK: Society for the Study of Artificial Intelligence and the Simulation of Behaviour), pp. 37-42. [pdf]
- J.L. McKinstry, G.M. Edelman, J.L. Krichmar (2006) A cerebellar model for predictive motor control tested in a brain-based device Proc Natl Acad Sci USA, 103: 3387-3392. [pdf]
- J.G. Fleischer, B. Szatmáry, D.B. Hutson, D.A. Moore,
J.A. Snook, G.M. Edelman, J.L. Krichmar (2006) A neurally
controlled robot competes and cooperates with humans in Segway
Sooccer. In Proceedings of the IEEE International Conference on
Robotics and Automation, to appear. [pdf]
- B. Szatmáry, J.G. Fleischer, D.B. Hutson, D.A. Moore,
J.A. Snook, G.M. Edelman, J.L. Krichmar (2006) A Segway-based
human-robot soccer team. In Proceedings of the IEEE International
Conference on Robotics and Automation, to appear. [pdf]
2005
- Krichmar, J.L. and G.M. Edelman, (2005) Brain-Based Devices for the Study of Nervous Systems and the Development of Intelligent Machines. Artificial Life, 11(1-2): p. 63-78.[pdf].
- J.L. Krichmar, A.K. Seth, D.A. Nitz, J.G. Fleischer, G.M. Edelman (2005) Spatial navigation and causal analysis in a brain-based device modeling cortical-hippocampal interactions. Neuroinformatics, 3(3), pp. 197-222 [pdf].
- A.K. Seth, O. Sporns, J.L. Krichmar (2005) Neurorobotic models in neuroscience and neuroinformatics. Neuroinformatics 3(3), pp. 167-170 [pdf].
- J.L. Krichmar, D. A. Nitz, J.A. Gally, and G. M. Edelman (2005).
Characterizing functional hippocampal pathways in a brain-based device
as it solves a spatial memory task. Proc Natl Acad Sci USA, 102:
2111-2116. [Abstract]
[Full Text]
[Figures
Only] [[pdf]]
[Supporting
Information]
2004
- A.K. Seth, J.L. McKinstry, G.M. Edelman, and J.L. Krichmar (2004).
Visual binding through reentrant connectivity and dynamic
synchronization in a brain-based device. Cerebral Cortex, 14:11,
1185-99. Article is reprinted, with permission, from Oxford University
Press. [full
text/pdf]
- A.K. Seth, J.L. McKinstry, G.M. Edelman, J.L. Krichmar (2004) Texture
discrimination by an autonomous mobile brain-based device with
whiskers. Proceedings of the 2004 IEEE International Conference on
Robotics & Automation (ICRA), New Orleans, LA, April 2004.
pp. 4925-4930 [[pdf]].
2003
- J.L. Krichmar, G.M. Edelman (2003). Brain-Based Devices: Intelligent
Systems Based on Principles of the Nervous System. In IEEE/RSJ
International Conference on Intelligent Robots and Systems (IROS). pp.
940-945: Las Vegas, NV, [[pdf]].
2002
- J.L. Krichmar, G.M. Edelman, (2002) Machine Psychology: Autonomous
Behavior, Perceptual Categorization and Conditioning in a Brain-Based
Device, Cerebral Cortex 12:818-830.[[pdf]].
Article is reprinted, with permission, from Oxford University Press.
- J.L. Krichmar, J.A. Snook (2002) A Neural Approach to Adaptive Behavior
and Multi-Sensor Action Selection in a Mobile Device, Proceedings of
the 2002 IEEE International Conference on Robotics and Automation,
3864-3869 [[pdf]].
2000
- J.L. Krichmar, J.A. Snook, G.M. Edelman, O. Sporns, (2000)
Experience-dependent Perceptual Categorization in a Behaving Real-World
Device, From Animals to Animats 6: Proceedings of the Sixth
International Conference on the Simulation of Adaptive Behavior, MIT
Press [[pdf]].
Article is reprinted, with permission, from From
Animals To Animats 6, published by the MIT Press.
Contact Jeff Krichmar for more information.
|
|
|
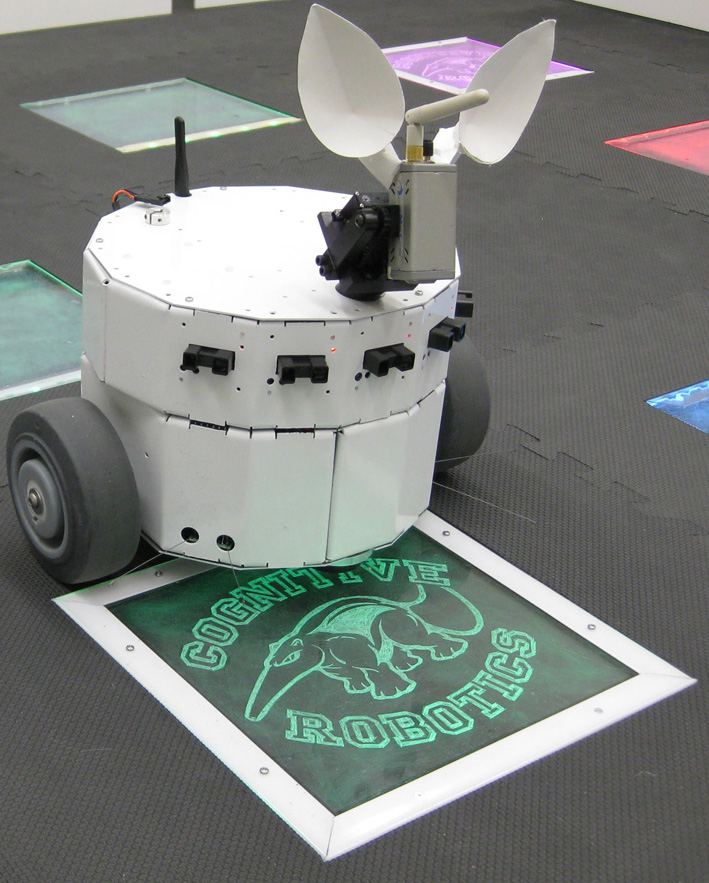
|